In my previous post, we learnt how to bake a data science cake (or not) the right way. However, if baking a cake isn’t your cup of tea/coffee or if you really want to scale up that baking business, you’re in the right place because we’re moving to retail. Retail is an interesting nut to have but a tough nut to crack and if that reminds you of walnuts, well, you will be interested in what we have to show (and tell). While we were busy baking a cake in California, our data scientist’s sister was practicing her lean, mean, machine learning skills up north. You know what they say about twins and now let’s see how that story unfolds.
Walnut Co. is a retail shop that originated in downtown Personhattan with a very young and ambitious Mr. Lobias Tutke. Originally planned as an online retail store for walnuts, owing to Mr. Tutke’s Californian ancestry, he figured that if he could sell walnuts and grab a big margin, why can’t he try selling other types of nuts? Eventually, why can’t he try selling all sorts of products through his retail website? He could become the Amazon.com for nuts or Amazon.com for, well, anything.
After having converted this excellent idea into reality and having operated since 2014, Mr. Tutke realized that his cost of operations has increased whereas his margins have remained low. His online marketing campaigns were likely a big hit but that didn’t necessarily trickle down to more converted customers or an exceptional hike in revenue. At this juncture, without risking any losses, he turned to Dr. Kaphne Doller – our data scientist extraordinaire.
At Walnut Co., with around 2 years of transactional data, our data scientist came across what could only be defined as the modern equivalent of a treasure chest filled to the brim with gold coins. “Data is the new oil,” as rightly termed by, well, every Wall Street Journal article ever and it could not have been more true in our case. This allowed us to dive into the realm of descriptive analytics – a branch of analytics defined by its ability to extract and summarize relevant information based on past data at a higher level of abstraction. This enabled Dr. Doller to capture a visual snapshot of the company’s past performance. Upon analysis, she found that although a lot of investment was made in physical advertisements (billboard, et al.), the positive correlation between those investments and the company’s revenue was low. Investments in social media marketing, however, yielded better returns and a stronger positive correlation with revenue. Additionally, the analysis provided reports on the status of certain inventory which have become a sunk cost to the company due to lack of demand in the market. However nifty, this form of analysis is known as descriptive analytics and it is usually only the first step in a data-driven solution engineering which reports information about a company at a high level. To dive deeper, Dr. Doller moved on to investigate what caused the revenue to dip.
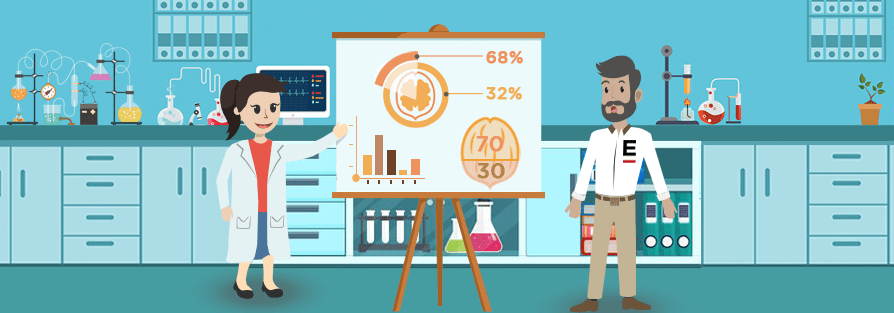
Enter diagnostic analytics – a much-needed addendum to the plain old descriptive analytics with the sole purpose of providing reasons for why something did or did not happen. The idea here is to compare multiple relationships between factors such as a Facebook marketing campaign asking your immediate friends to buy walnuts versus a LinkedIn campaign to reach out to relevant connections – to determine the reasons behind the success or failure of one campaign over another. Dr. Doller here, equipped with the knowledge of A/B testing, decided to compare different types of marketing campaigns to see what resulted in an increase in revenue through higher conversion rates, clicks, and other important KPIs. Upon investigation, she found that not only was a bulk of money invested in these campaigns but also neither of them generated enough conversions to drive revenue in 2015-16. This investment faux-pas set back Walnut Co. by a lot and was one of the key contributors to lower revenue for the company. Once informed, Dr. Doller communicated her findings to Mr. Tutke and explained the importance of performing A/B tests prior to investing that kind of money in future campaigns.
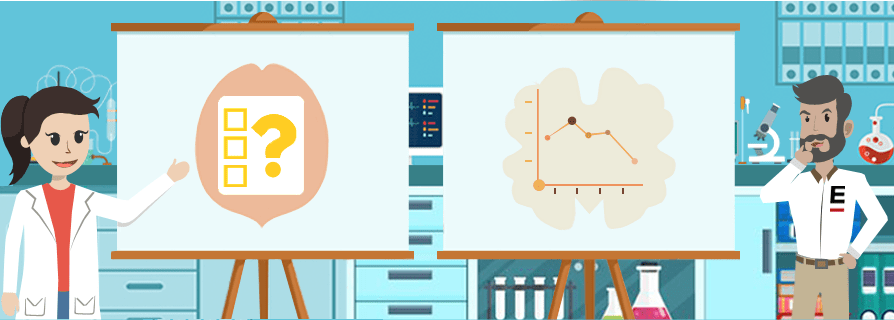
You’d imagine that would solve our problem but we are nowhere close to the entire solution. At this point we know how the business is performing, and what caused a temporary revenue crunch – what we don’t know is how to predict future performance with our current data set. This brings us to predictive analytics, a form of analytics dedicated to providing future insights on the basis of historical data. This type of analytics involves a number of statistical methods and machine learning algorithms to make predictions with a certain degree of confidence about future trends. Based on current consumer behavior and trends in the market, Dr. Doller decided to forecast the demand for a number of products to determine inventory status for Walnut Co. She worked out some statistical models, primarily time series models and regression models, and let Mr. Tutke know that cashew nuts (and not walnuts) would be the next big thing with a demand that would increase its market price by about 27%.
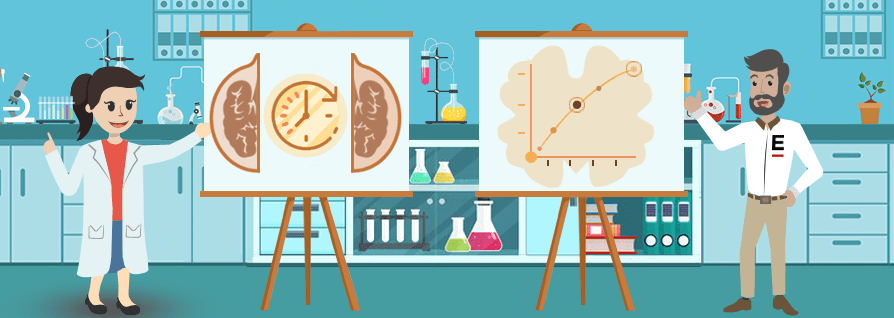
At its final stage, Dr. Doller implemented prescriptive analytics to help Mr. Tutke drive his business decisions. Now that we are aware of the overall business standing, the cause for sudden revenue loss and the future trends that are likely to reign over the area of retail and products for the upcoming year – we need to find a way to adapt in order to retain customers and make more money. Prescriptive analytics takes predictive analytics a step further by not only predicting changes but also recommending methods of combating those changes (if they are not helpful to the business) as well as ways to amend the current processes prevalent in the company.
In this instance, Dr. Doller knew that the prices of cashew nuts were going to go up based on her predictive model. This answered the two economic questions of how much to stock and how much to sell the nuts for. She built a model to predict both cashew nut stocks and selling price; notifying Mr. Tutke to purchase at least 100kg of cashew nuts and to sell them at 30% more than its previous running price. Thus, this prescriptive analytics solution not only suggested the amount to stock but also aided in the process of setting the price point for a particular product.
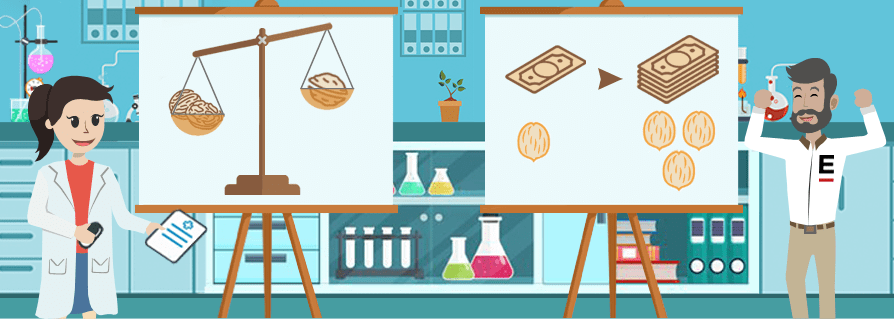
A strong business is a business that knows how to keep its bottom line healthy, and thanks to Dr. Doller, Walnut Co. had its day and seized it too.
Coverage in TimesTech
Great post, I really enjoyed reading it. You explained everything so clearly and made it easy to understand. Looking forward to more posts like this, this informations are very helpful for me.
<a href="https://cognitec.in/">BEST DATA SCIENCE AND DATA ANALYTICS COURSE IN CHENNAI</a>
<a href="https://cognitec.in/">DIPLOMA COURSE IN DATA ANALYTICS</a>
<a href="https://cognitec.in/">DATA ANALYTICS COURSE ONLINE</a>
<a href="https://cognitec.in/">DATA ANALYTICS CERTIFICATION COURSES IN CHENNAI</a>
<a href="https://cognitec.in/">DATA ANALYSIS COURSES FOR BEGINNERS IN CHENNAI</a>
<a href="https://cognitec.in/">BEST DATA ANALYTICS CERTIFICATION COURSE IN CHENNAI</a>
<a href="https://cognitec.in/">DATA ANALYTICS COURSE NEAR ME</a>
<a href="https://cognitec.in/">MASTERS IN DATA SCIENCE AND ARTIFICIAL INTELLIGENCE IN CHENNAI</a>
<a href="https://cognitec.in/">MSC ARTIFICIAL INTELLIGENCE AND DATA SCIENCE ONLINE</a>
<a href="https://cognitec.in/">MASTER DATA SCIENCE AND ARTIFICIAL INTELLIGENCE</a>
<a href="https://cognitec.in/">ARTIFICIAL INTELLIGENCE POSTGRADUATE COURSES</a>
<a href="https://cognitec.in/">MASTER OF SCIENCE IN DATA SCIENCE AND…